When it comes to translation, one common topic is centered around machine translation (MT), which refers to the use of software to automate translation. Machine translation underwent a quantum leap about five years ago with the advent of neural machine translation (NMT). For example, in a 2016 study, Google stated that their NMT system (GNMT) reduced translation errors by 60% compared to their previous phrase-based statistical MT system. The automated metrics that score machine translation by comparing it to human translations demonstrate that this jump in quality is significant (see Figure 1).
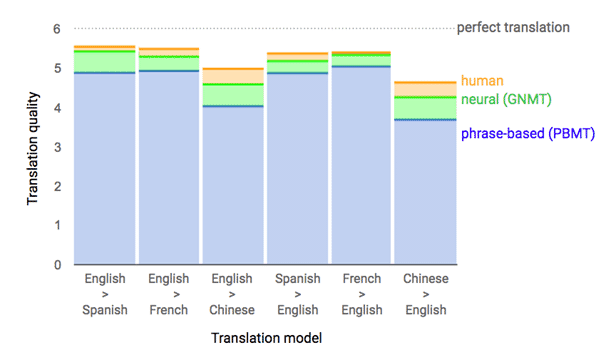
Figure 1.
With these advancements in mind, it's appropriate to discuss the ways in which machine translation can be used in a professional environment.
Considerations When Using Machine Translation
Before listing the options that integrate MT into the translation workflow, it's useful to highlight several considerations that will affect which option is best for you and your situation.
1. Language pairs: MT is much better in languages where the amount of available translated data is significant. It's also the case that different MT systems perform better on certain language pairs. For example, DeepL outperforms almost every system (see Figure 2), but doesn't support as many languages as Google or Microsoft. If your project is translated from or into European languages, then the NMT systems available will be significantly better than if you’re translating into Asian or Middle-Eastern languages.
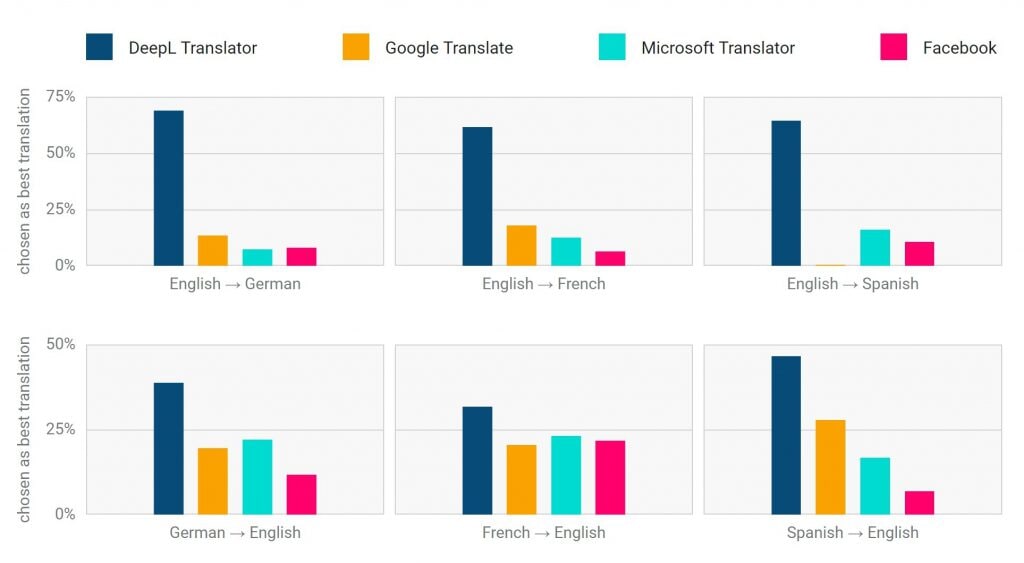
Figure 2.
2. Data privacy: Most MT providers are aware that data privacy is an issue, and attempt to solve or at least tackle the issues with data security agreements and on-premise options. While selecting an MT provider, consider your project and read their privacy policies with the sensitivity of your content in mind.
3. Domain: The subject matter of the translation project will also affect the quality and effectiveness of the NMT system. If your domain is generic or highly available online (e.g. software documentation, governmental), the translation quality will be significantly better. If you’re translating content in a smaller niche domain (e.g. oceanography) or a highly sensitive domain (e.g. health care), the relatively small amount of publicly available training data will compromise quality.
How to Leverage Machine Translation
Understanding those factors, you can now better decide how machine translation can be used in your project. Here are some ways that MT can be used in your translation workflow.
1. Create a Baseline for Content: While most content needs to be as perfectly translated as possible, there are use cases where something would be better than nothing. In scenarios where the alternative is no translation at all, MT can be useful. If your documentation is only for in-house use, starting with a MT version can also help indicate if a professional translation is necessary.
2. Pre-translate with MT: In this case, either you can pre-translate your content using an MT system inside of MadCap Lingo or your CAT tool of choice, then send it to your language service provider (LSP). If you don't use MadCap Lingo or any CAT tool, you can also ask your LSP about pre-translation options, what pricing is for MT post-editing, and which MT provider they use.
3. Train MT system: Some NMT systems, just like previous statistical models, can be improved given quality and domain-specific training data (see Figure 3 below). If you have a large Translation Memory available to you, you can investigate this option. Some MT providers offer customizable NMT engines. However, training an engine takes time, comes at an additional cost, and requires some knowledge about translation standards, so talk to your LSP to determine if it's a worthwhile investment for you.
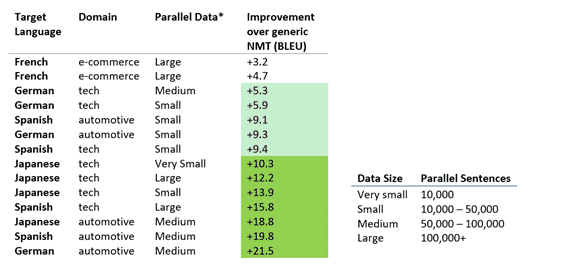
Figure 3.
4. Use as an internal resource for translators: Translators often use MT as a resource. If your company has an in-house translation team, or if you have a good relationship with your LSP, coordinate with your translators to reference the same MT system. This can help throughput and provide consistency in your translation efforts.
Although improvements and advancements in machine translation have made MT a worthwhile component of the translation workflow, it’s still vital to maintain a language professional in the loop, as they provide accuracy and valuable context in the native language. While an ideal workflow consists of a balance between human and machine translation, it’s worth evaluating how MT can be used to improve your translation efforts. Reach out to your language provider or translation team to discuss the possibilities, and if you don't have a trusted LSP, reach out to MadTranslations and let them help you decide how to leverage MT.
Keep an eye out for our follow-up blog post, which discusses machine translation from an LSP’s perspective, and how translators are using MT.